
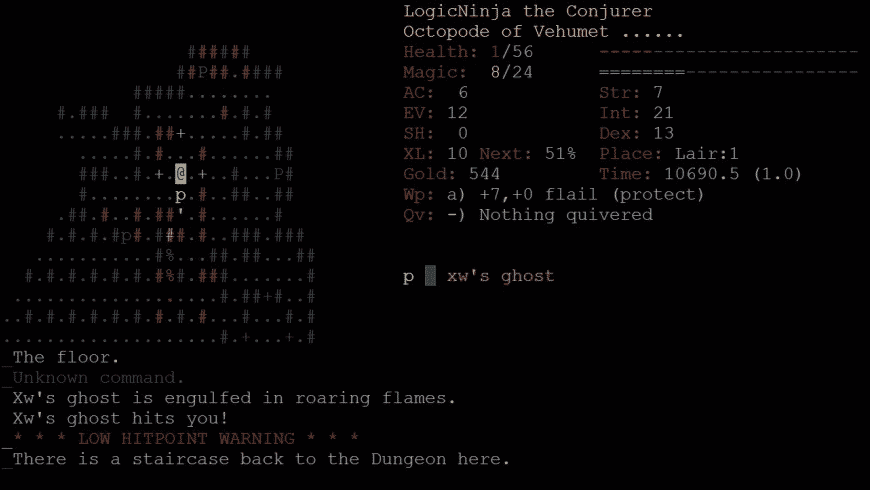
First off, there are twenty six player character races to choose from including many rarely found in commercial computer games. Note: Always make sure the Num Lock key is OFF on your keyboard when you play CRAWL.ġ.
GETTING GOOD AT DUNGEON CRAWL STONE SOUP ZIP FILE
Never played a roguelike before? Follow along with this overview, skim the documentation included in the CRAWL zip file and you'll be all set. The object of CRAWL is to find and steal a great mystical Orb guarded by demons, and far worse entities, in the dangerous magic realm of Zot, a feat few have achieved without cheating.ĬRAWL features ".Large, highly random dungeon settings huge range of races/classes to choose from comprehensive skill system complex magic system with about 140 spells hundreds of monsters multiple dungeon branches and gates to other planes a dozen religions to choose from random artifacts, mutations, vaults, water, lava, clouds, yaks and many more weird things!" It's a roguelike: a randomized underground adventure simulation in the style of Rogue, NetHack and Dungeons & Dragons, yet offers its own twists and a unique magical fantasy world to explore. In Knowledge Extraction from Games Workshop at AAAI-18, 2018.CRAWL, aka Dungeon Crawl, by Linley Henzell and the CRAWL development team, is a free, easy to learn yet difficult to master fantasy role playing game. Starcraft ii: a new challenge for reinforcement learning.Ĭoncept-aware feature extraction for knowledge transfer in Vezhnevets, Michelle Yeo, Alireza Makhzani, Heinrich Küttler, John Oriol Vinyals, Timo Ewalds, Sergey Bartunov, Petko Georgiev, Alexander Sasha Yuandong Tian, Qucheng Gong, Wenling Shang, Yuxin Wu, and C. Lawrence Zitnick.Įlf: An extensive, lightweight and flexible research platform forĪdvances in Neural Information Processing Systems (NIPS), 2017. Kurt Steinkraus and Leslie Pack Kaelbling.Ĭombining dynamic abstractions in large mdps. In Proceedings of the 25th AAAI Conference on Artificial Preferred explanations: Theory and generation via planning. In Proceedings of the 26th AAAI Conference on Artificial IEEE Transactions on Computational Intelligence and AI inĭungeon crawl stone soup tournament results v 0.18. Santiago Ontanón, Gabriel Synnaeve, Alberto Uriarte, Florian Richoux, DavidĪ survey of real-time strategy game ai research and competition in Pddl-the planning domain definition language. AAAI Press, 2017.ĭrew McDermott, Malik Ghallab, Adele Howe, Craig Knoblock, Ashwin Ram, Manuela In Proceedings of the 31st AAAI Conference on Artificial Pat Langley, Ben Meadows, Mohan Sridharan, and Dongkyu Choi.Įxplainable agency for intelligent autonomous systems. The malmo platform for artificial intelligence experimentation.
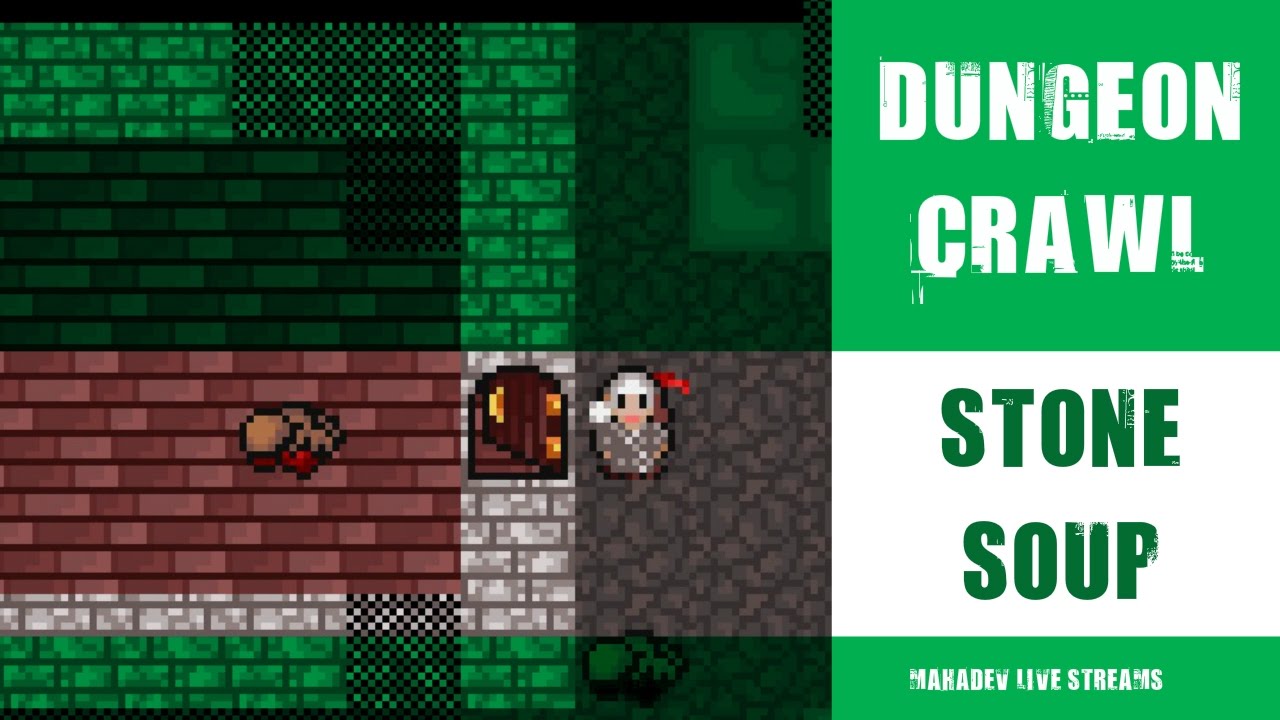
Matthew Johnson, Katja Hofmann, Tim Hutton, and David Bignell.
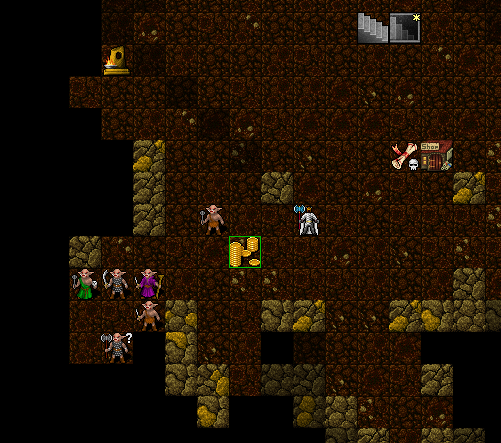
In Explainable Artificial Intelligence: Papers from the IJCAI Maria Fox, Derek Long, and Daniele Magazzeni. In Proceedings of the 26th International Joint Conference onĪrtificial Intelligence, pages 156–163, 2017. Plan explanations as model reconciliation: Moving beyond explanation T. Chakraborti, S. Sreedharan, Y. Zhang, and S. Kambhampati. In Proceedings of Multikonferenz Wirtschaftsinformatik, pages Verbal plan explanations for hybrid planning. Julien Bidot, Susanne Biundo, Tobias Heinroth, Wolfgang Minker, Florian For example, research on Explainable Planning has focused on helping humans to understand a plan produced by the planner (e.g., ), on reconciling the models of agents and humans (e.g., ), and on explaining why a particular action was chosen by a planner rather than a different one (e.g. While XAI research on data-driven ML is well-motivated, AI Planning is well placed to address the challenges of transparency and explainability in a broad range of interactive AI systems. Most of these efforts have focused on providing transparency to the decision making of machine learning (ML) systems in general, and deep networks more specificallyExceptions, for example, include the broader intent of the Workshops on XAI at IJCAI-17 and IJCAI-18. The interpretability of AI systems has been a popular topic of workshops and related events since 2016, and in 2017 DARPA launched the Explainable AI (XAI) Program. Explainable planning and goal reasoning agents:
